the Creative Commons Attribution 4.0 License.
the Creative Commons Attribution 4.0 License.
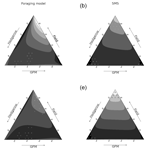
Keep your enemies closer: enhancing biological control through individual movement rules to retain natural enemies inside the field
Thomas Delattre
Blanche Collard
Claire Lavigne
Biological control of pests aims at lowering population levels of pest species by favouring natural enemies, in order to reduce the use of pesticides. The movement behaviour of natural enemies is decisive in the success of biological control: when low habitat quality hinders the diffusion of natural enemies from the border, the density of natural enemies may frequently be heterogeneous inside agricultural plots.
We hypothesise that the specific relationship between habitat quality and movement behaviour may allow the improvement of biological control by means of a careful allocation of habitat qualities inside and around the plot.
We used three tested individual-based movement models, with different levels of complexity ranging from simple cell-to-cell movements to complex strategies including the sinuosity of the path, boundary crossings, perceptual range, and directional persistence. We used the models to explore how the manipulation of habitat quality may allow significant improvements to the residence time of natural enemies inside the field. We suggest that existing field designs are generally inadequate to retain natural enemies. Mechanistic explanations leading to the highest and lowest residence times are used to draw specific management recommendations.
- Article
(740 KB) -
Supplement
(901 KB) - BibTeX
- EndNote
Detrimental effects of pesticides on biodiversity and human health require alternative pest control methods (Human Rights Council, 2017). Biological control of pests aims at reducing population levels of pest species by favouring indigenous natural enemies, or by introducing new ones. The biological control service results from a number of ecological processes, each of which needs to be adequately addressed (Root-Bernstein and Jaksic, 2017). One of these processes is the movement of individuals from pests and natural enemies, which affects their spatio-temporal co-occurrence. Indeed, the movement ability and behaviour of potential natural enemies are regularly discussed as a risk for the success of conservation biological control (Arrignon et al., 2007; Barbosa, 1998; Kremen et al., 2007; Pulido and Berthold, 2010; Simberloff and Stiling, 1996).
Agricultural plots are rarely designed to reproduce the characteristics of natural ecosystems (Malézieux et al., 2009). Being monospecific and often based on non-native species, they are perceived as low-quality habitats by an important proportion of indigenous species (Altieri and Policy, 2001; Wäckers et al., 2005). Theory predicts and empirical evidence indicates that individuals from most taxa tend to avoid entering hostile habitats, and reduce time spent in it, when forced to leave their habitats (Van Dyck and Baguette, 2005). Expectedly, the density of natural enemies in fields often decreases steeply with distance to the field borders (Al Hassan et al., 2013; Lys et al., 1994) and hedgerows (Coombes and Sothertons, 1986; Lys et al., 1994). Habitat diversification is a frequently advocated strategy to favour conservation biological control, with a focus on species composition but not on its spatial distribution (Barbosa, 1998; Ratnadass et al., 2012). The amount of semi-natural areas tends to lower the abundance of in-field pests, but there is no clear direction in relationships between pest control and landscape composition (Veres et al., 2013). Here, we explore how the specific relationship between the habitat quality of landscape elements surrounding the field and the movement behaviour of target species may affect biological control.
Potential markers indicating that field and landscape characteristics tend to favour biological control would be an increase in the time spent by natural enemies inside the field and if that time is homogeneous over the field area. Indeed, increasing the time spent by natural enemies inside, the plots should increase the probability of detecting preys, or at least detecting clues that would trigger a foraging sequence (Börger et al., 2008; Mitchell and Powell, 2004).
The behavioural mechanisms of movement are strongly affected by the quality of habitat as it is perceived by the moving individual. Leaving a resource patch is favoured by low contrasts at habitat boundaries; movement paths are less sinuous in bad-quality habitats; good habitat patches are more likely to be chosen as destinations. Those simple rules are strongly supported by empirical evidence (Clobert et al., 2001), are widely shared across taxa (Van Dyck and Baguette, 2005), and therefore provide a good basis to predict movements of natural enemies inside agricultural fields.
It is frequently advocated that landscape composition could contribute to sustainable pest control, either by directly impacting pest abundance or indirectly affecting its natural enemies (Veres et al., 2013). In this contribution, we used a modelling approach to explore how the ground cover quality of landscape elements may be manipulated to increase the residence time of natural enemies inside the field. We started from a typical situation with fields surrounded by hedgerows and grassy field margins, and we explored how the habitat quality of these three landscape elements affected the residence time of individuals. For simplicity, we focused on natural enemies originating from hedgerows because semi-permanent habitats are frequently advocated as refuges from the perturbations of farming practices (Griffiths et al., 2008). However, the results and reasoning may easily be adapted to study natural enemies originating from other landscape elements, as well as pests.
We used individual-based models, one of the best approaches to predict spatial patterns emerging from interactions between individual behaviours and their environment (Dover and Fry, 2001; Grimm and Railsback, 2005; Haddad, 1999). We compared three tested movement models in order to increase the robustness and genericity of our results.
We tested a simple foraging-like model in which individuals choose the best cell around them at each time step, without preferential directionality, perceptual range, or context-dependent strategy. This model serves as a baseline for the interpretation of the two following more complex models.
We tested SMS (stochastic movement simulator; Coulon et al., 2015), an extension of the above model with the addition of a perceptual range (in which the “best” cell is chosen based on a weighted lottery) and a directional persistence.
We tested the routine and direct movements (RDM) model (Delattre et al., 2010b; Kindlmann et al., 2004), in which boundary crossing depends on boundary contrast, and the sinuosity of the path is influenced by the quality of the habitat in which movement occurs.
We hypothesise that focusing on how the distribution of habitats influences the movement behaviour of natural enemies may facilitate the design of “suppressive” agricultural landscapes (Veres et al., 2013). We used an individual-based model (IBM) approach to
-
test if the manipulation of habitat may increase the residence time of natural enemies in the field,
-
explore a set of specific scenarios with significant impact on residence time or particular interest regarding existing field situations,
-
understand which behavioural mechanism caused the observed effect,
-
draw recommendations related to the management of the field and its immediate vicinity.
2.1 The landscape
Our virtual landscapes were designed as simple mosaics of agricultural fields separated by hedgerows and grassy field margins (GFMs). Such patterns are typically found in western Europe where the common agricultural policy (CAP) provides incentives to farmers implementing 5–10 m width strips of grassland along field borders (Ernoult et al., 2013; Kleijn et al., 2006). This landscape configuration provides an interesting illustration to our question because
-
hedgerows are frequently advocated as sources of natural enemies for agricultural fields;
-
the value of GFMs for biodiversity and ecosystem services other than water is still a matter of debate (Delattre et al., 2013; Ernoult et al., 2013; Al Hassan et al., 2013; Kleijn et al., 2006);
-
the addition of GFMs allows investigation of more complex interactions among habitat qualities than a simple binomial landscape;
-
hedgerows and GFMs allow investigation of potential counteracting effects such as corridors or barriers.
Field shapes and patterns were obtained using a method similar to a T tessellation (Papaïx et al., 2014) that consists of seeding the landscape with a defined number of randomly distributed seeds, each of which is a departure point for three edges that eventually form a rectangle (Fig. 1). This method allowed probabilistic control on the number of polygons and their size and shape while exploring a diversity of spatial distributions of field shapes and sizes (Fig. 1). In order to focus on habitat quality, the patch density was kept constant to maintain a stable landscape structure throughout the simulations (see Supplement S2 for the effect of patch density) and we altered only habitat quality for each landscape element (with an interval of 5, from 1 to 99, from hostile to favourable). Each combination of habitat qualities was simulated 30 times to account for the variations in field shapes.
The landscape was a 500-pixel-wide square treated as a torus and was composed of 10 to 12 fields surrounded by 4-pixel-wide hedgerows and 5-pixel-wide GFMs (similar to a typical bocage landscape; Burel et al., 1998; Thenail and Baudry, 2004). Although the field–GFM–hedgerow trio is used as an example for clarity, the structure could apply to fields surrounded by other types of borders.
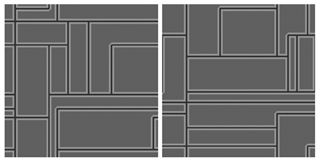
Figure 1Two examples of artificial landscape structures generated with 12 seeds. Agricultural fields (dark grey) are separated by grassy field margins (light grey) and surrounding hedgerows (black).
For simplicity, the habitat quality of all three habitats always sums to 100. This reflects practical and budget constraints that prevent unlimited improvement of all habitat types in the field.
2.2 The models
2.2.1 Initialisation and common parameters
All three movement models were initialised with the same common parameters, with the exception of movement parameters that are described in the specific model sections below. In each movement model, 2000 individuals were randomly distributed along hedgerows to simulate the common assumption that hedgerows are sources of natural enemies. Individuals were initialised with an energy pool of 500 energy units, representing their intrinsic initial movement ability. At each time step, the remaining energy pool was decremented by , where q represents habitat quality of the current cell. This mechanism allowed us to mimic the direct and indirect costs of movement (Bonte et al., 2012) that are high in hostile habitats and low in favourable habitats. The habitat sensitivity parameter (Table 1) was used to alter the effect of habitat quality on movement cost, as a proxy of interspecific differences in habitat sensitivity. A random value [] was added to the pixel cost with a probability equal to the habitat sensitivity of the species/100 (i.e. adding noise around the cost value). The costs of diagonal and orthogonal moves were identical. The simulation ended when all individuals had depleted their energy pool.
2.2.2 Outputs
All three models simulated the paths followed by virtual individuals depending on the distribution of habitat qualities, translated as a map of cost values. Here, the descriptor of the resulting path that we chose to focus on is the residence time in the fields. Mean field residence time of a landscape was measured as the mean time a field pixel was occupied by an individual (hereafter, it is given in model time step per pixel) and was considered to be an important component of biological control. Variance of this measure was estimated over all field pixels in a landscape, meaning that a high variance would indicate a high spatial heterogeneity between field pixels in their residence time (e.g. if the centres of fields were avoided). Spatial variation in the field frequentation was also illustrated by the proportion of unvisited field pixels, i.e. pixels that were not crossed by any individual during the simulation.
2.2.3 Model 1: foraging model
The foraging model was the simplest of the three models, and was intended to illustrate a foraging-like movement with as few assumptions as possible. At each time step, individuals compared the habitat quality of eight neighbouring cells and identified the best one. In order to compare different species' responses to sets of habitat qualities, we added a habitat sensitivity parameter that illustrates interspecific variability in sensitivity to habitat quality (i.e. generalist vs. specialist species). The probability that a random cell was chosen instead of the better one was inversely proportional to the habitat sensitivity of the species (i.e. a species with a low habitat sensitivity would have a higher probability to ignore the better cells and engage in a random walk). A complete description of the foraging model using the ODD (overview, design concept, and details) protocol (Grimm et al., 2006, 2010) is provided in the Supplement.
2.2.4 Model 2: stochastic movement simulator
SMS is a model developed by Palmer et al. (2011) that allows us to reliably estimate the reaction of various species to landscape characteristics (Coulon et al., 2015; Palmer et al., 2011). It is based on only two necessary parameters, one controlling the degree of correlation in simulated movements (directional persistence) and one describing the distance at which animals can detect and respond to landscape properties (perceptual range). The sensitivity of a species to habitat quality was illustrated by different values of directional persistence and perceptual range (Table 1). An insensitive species with a high directional persistence and a high perceptual range is more likely to concentrate on areas with better habitat qualities, even if they are separated by hostile habitats. An extensive description of the SMS model is provided in Bocedi et al. (2014) and Coulon et al. (2015).
2.2.5 Model3: RDM model
The routine and direct movements (RDM) model is a model developed by Kindlmann et al. (2004) and Delattre et al. (2010b) to simulate the movement behaviour of the butterfly Maniola jurtina L. (Nymphalidae) (Kindlmann et al., 2005) in agricultural landscapes. It is a good example of a model illustrating species with a precise definition of habitat patches and high reaction to boundaries. It is grounded on the principles developed by Van Dyck and Baguette (2005) with a context-dependent movement behaviour, including linear “direct” movements occurring in hostile habitats and sinuous “routine” movements occurring in favourable habitat patches. Emigration from a patch is proportional to the contrast between habitats on each side of a boundary. Like in the foraging model, the habitat sensitivity parameter was added in order to compare different scenarios with different species' response to landscape. The effect of habitat quality on the sinuosity of the path and on the probability to cross a boundary are proportional to the habitat sensitivity of the species: an insensitive species will be more likely to ignore the current habitat quality when defining its path sinuosity and to ignore the contrast of a boundary when deciding if it is to cross it. A complete description of the RDM model using the ODD protocol (Grimm et al., 2006, 2010) is provided in the Supplement.
2.2.6 Expected differences between models
The foraging model was used as a null hypothesis, simulating species without perceptual range or known strategy to use space. The only behaviour expected from this model was that individuals should tend to avoid hostile habitats and tend to stay in nearby favourable habitats.
SMS integrated the basic behaviour of the foraging model while adding two parameters. Directional persistence introduced a tendency to cross good and bad habitats, much like a conservation of momentum, which should result in individuals spreading more evenly in the landscape and a lower effect of contrasted landscape elements such as barriers. Perceptual range provided the individual with information about habitat quality beyond the eight neighbours used in the foraging and the RDM models. Perceptual range should result in individuals being able to cross obstacles more easily than in other models (if perceptual range >1) and in a higher tendency to concentrate into the best habitats.
The RDM model integrated a sensitivity to boundaries between different habitats that should result in individuals staying in good habitats by avoiding movement, and a sinuosity parameter that should result in lower time spent in hostile habitats during movement.
Of all three models, the foraging model should predict that individuals stay closer to favourable habitats, with short movements concluded by a quick return to favourable habitats. SMS should allow for higher emancipation from local conditions resulting in both higher probabilities to leave habitats and time spent in hostile habitats (because the geometry of the paths does not adapt when crossing bad habitats). In an intermediate manner, the RDM model should allow for low probabilities to leave habitats, similar to the foraging model, but higher coverage of hostile habitats due to a shift to directional movements.
2.2.7 The hypothetical species
We used two hypothetical species to illustrate the sensitivity of the three models to differences in habitat sensitivity. An “insensitive species” with a movement behaviour that allowed individuals to free themselves from local habitat conditions to reach a another region of the landscape more easily: in the foraging model and the RDM model this species was characterised by a lower value of the sensitivity to habitat quality parameter (Table 1); in SMS, that species was characterised by a higher directional persistence and a higher perceptual range. Conversely, the “sensitive species” was characterised by a movement behaviour that depended more strongly on local conditions (Table 1) with a higher sensitivity to habitat (foraging and RDM models) and lower directional persistence and perceptual range (SMS). Each simulation of each model was performed with 2000 individuals that moved until their energy pool was depleted.
2.3 Data analysis
The effects of landscape parameters in determining fields' visits were examined by fitting the simulated residence times in statistical models having each habitat quality and all possible first-order interactions among them as explanatory variables. The proportion of unvisited pixels was fitted to a generalised linear model incorporating a logit link function and a binomial error term and the same explanatory variables. The relative importance of habitat qualities and their interactions was assessed by McFadden's pseudo r squared (Long, 1997). Ternary diagrams were produced using the ggtern R package (Hamilton, 2017) and the R software (R Core Team, 2011).
3.1 Residence time
3.1.1 Effect of habitat quality
The quality of fields had the greatest effect on mean field residence time (pr2>0.92) in SMS and RDM models, for both virtual species (Fig. 2). It had a substantial effect on residence time in the foraging model too (sensitive species: pr2=0.28; insensitive species: pr2=0.57), and there was also a notable interaction with the hedgerow quality in the sensitive species (pr2=0.15). Residence times varied between 0.25 time steps (ts) and 1.83 ts in the RDM model, between 0.02 and 2.18 ts in SMS, and between 0.0002 and 1.86 ts in the foraging model (Fig. 4).
The RDM model predicted higher residence times for high field qualities and low GFM and hedgerow qualities, while high-quality GFMs with low-quality fields and hedgerows brought the lowest residence times. Overall, field quality had a positive effect, hedgerow quality had a negative effect, and GFM quality had the most negative effect.
SMS also predicted higher residence times at high field qualities and low GFM and hedgerow qualities, but the lowest residence times were observed for both higher values of hedgerow quality (with low GFM and field qualities) and higher values of GFM quality (with low field and hedgerow qualities). Overall, field quality had a positive effect, GFM quality had a negative effect, and hedgerow quality had the most negative effect on residence times (pull effect).
The foraging model also predicted the highest residence times at higher field qualities, although it was more substantially mediated by GFM habitat quality, with low residence times at low GFM quality (even when field quality was high). The lowest residence times were observed at low GFM quality, low field quality, and high border quality.
3.1.2 Effect of model and species
Mean field residence times were low (<0.9 ts) in every model and for both virtual species (Fig. 4) although the effect of habitat configurations allowed residence times of up to 2 ts. The RDM model predicted the highest residence time, followed by SMS and the foraging model (Fig. 4). The insensitive species showed a higher residence time than the sensitive species in both the foraging model and SMS, but not in the RDM model. The sensitive species showed a particularly low residence time (Fig. 4) in the foraging model.
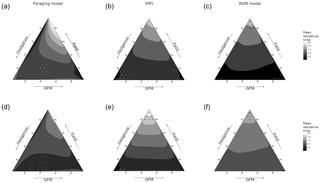
Figure 2Mean field residence time (in time steps, ts) as a function of species, model, and habitat quality of the three habitat types (fields, hedgerows, grassy field margins). The sides of the triangle are scaled in percentages of habitat quality of the three habitat types (0–100 %). (a, d) Foraging model. (b, e) Stochastic movement simulator. (c, f) Routine and direct movements model. (a–c) Species with high habitat sensitivity during movement. (d–f) Species with low habitat sensitivity. Surfaces are rendered using a cubic interpolation of ternary values. The corresponding actual values are illustrated by circles (lowest 5 % of values) and crosses (highest 5 % of values).
3.2 Spatial variation in residence time
3.2.1 Effect of habitat quality
The quality of fields had the greatest effect on the proportion of unvisited pixels (pr2>0.52) in SMS for both virtual species, and there was also a substantial interaction between hedgerow and GFM qualities (pr2>0.45). In the foraging model, the quality of fields also had the greatest effect on the proportion of unvisited pixels (pr2>0.45) for both virtual species, followed by hedgerow quality (pr2>0.25). The interaction between quality of fields and GFMs had the greatest effect on the proportion of unvisited pixels in the RDM model for both virtual species (insensitive species: pr2=0.20; sensitive species: pr2=0.35), followed by hedgerow quality for the insensitive species (pr2=0.17) and the interaction between hedgerow quality and field quality for the sensitive species (pr2=0.21). The proportion of unvisited pixels varied between 0.47 and 0.80 in the RDM model, between 0.25 and 0.99 in SMS, and between 0.57 and 0.99 in the foraging model (Fig. 4).
The RDM model predicted higher proportions of unvisited pixels at high field qualities and low GFM and hedgerow qualities, and also at high GFM quality and low qualities of hedgerows and fields. Overall, intermediate values of habitat qualities brought lower proportions of unvisited pixels, field quality had a positive effect, hedgerow quality had a negative effect, and GFM had the most negative effect.
SMS predicted lower proportions of unvisited pixels at high field qualities and low GFM and hedgerow qualities, but the lowest proportions of unvisited pixels were observed at both higher values of hedgerow quality (with low GFM and field quality) and higher values of GFM (with low field and hedgerow quality). Overall, field quality had a negative effect on the proportion of unvisited pixels, hedgerow quality had a positive effect, and the proportion of unvisited pixels was lower for intermediate values of GFM quality.
The foraging model also predicted the lowest proportions of unvisited pixels at higher field qualities, although it was more substantially mediated by GFM quality, with high proportions of unvisited pixels at low GFM quality (even when field quality was high). The higher proportions of unvisited pixels were observed at low GFM quality, low field quality, and high hedgerow quality.
3.2.2 Effect of model and species
The mean proportion of unvisited pixels was high (>0.6) in every model and for both virtual species. The RDM model predicted the lowest mean proportion of unvisited pixels, followed by SMS and the foraging model (Fig. 4b). There was a lower proportion of unvisited pixels in simulations of insensitive species, in both the foraging model and SMS, but not in the RDM model. The sensitive species showed particularly high proportions of unvisited pixels (Fig. 4) in the foraging model.
All models showed similar patterns of residence time and spatial variation in residence times, meaning that low residence time corresponded to high proportions of unvisited patches. The only exceptions were simulations of the RDM model for the insensitive species (Fig. 3), in which low residence times corresponded to either low proportions of unvisited patches (with high-quality hedgerows and low-quality GFMs and fields) or intermediate proportions of unvisited patches (with high-quality GFMs and low-quality fields and hedgerows).
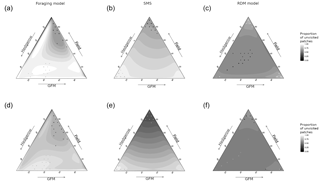
Figure 3Spatial variation in the field frequentation (proportion of unvisited field pixels) as a function of the model, species sensitivity to habitat, and habitat quality. The sides of the triangle are scaled in percentages of habitat quality of the three habitat types (0–100 %). (a, d) Foraging model. (b, e) Stochastic movement simulator. (c, f) Routine and direct movements model. (a–c) Species with high habitat sensitivity during movement. (d–f) Species with low habitat sensitivity. Surfaces are rendered using a cubic interpolation of ternary values. The corresponding actual values are illustrated by circles (lowest 5 % of values) and crosses (highest 5 % of values).
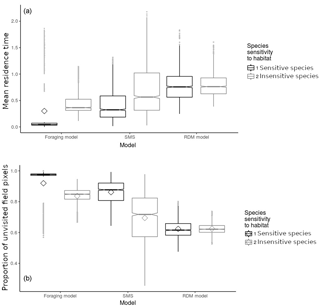
Figure 4Model and species comparison showing mean field residence time in time steps (ts) per pixel (a) and proportion of unvisited field pixels (b) as a function of model types (foraging model, routine and direct movements model, stochastic movement simulator) and species sensitivity to habitat quality. Diamonds represent the mean; notches represent the 95 % confidence interval around the median (which is represented by the horizontal junction between notches); vertical bars represent the 25th and 75th percentiles; grey dots represent outliers. Mean values and error indicators show the models' sensitivity to particular sets of habitat qualities (for each model and species, values are pooled and summarised from 30 repetitions of landscapes generated stochastically for each set of habitat qualities).
There is a growing recent interest in diversifying crop vegetation (Ratnadass et al., 2012) with a predominant focus on the composition of the diversity and its impacts on the trophic chain. We believe that there is substantial potential in combining this approach with the methods and concepts from landscape ecology, and in particular movement ecology. Acting on the spatial organisation of crop diversity and semi-natural habitats should help resolve the problems of spatial discrepancy between the regulation service and the population dynamics of targeted natural enemies, which hinders biological control approaches (Kremen et al., 2007). Here, we have presented a deliberately simple method, which uses the habitat quality of three habitat types (hedgerow, GFM, and field) as they are perceived by moving animals and models their impact on the frequentation of the field. Our approach compares three models that adequately describe movement in agricultural landscapes (Aviron et al., 2007; Coulon et al., 2015; Delattre et al., 2010b; Kindlmann et al., 2005; Palmer et al., 2011). Each model requires only one or two parameters (in addition to suitability maps) that are reasonably easy to document with field observational studies (Conradt et al., 2003; Delattre et al., 2010a, 2013; Stevens et al., 2003). A comprehensive study of the effect of plant species diversification on movement and frequentation of the field would require the consideration of an increase in structural diversity of the field, as well as a more heterogeneous mosaic of habitats including more than three habitat types (Fahrig et al., 2010; Vasseur et al., 2013). However, the complexity of such a system would make it difficult to disentangle the numerous interactions at play with a single approach, which is why we chose to focus on habitat qualities in this first attempt. Later, we explain the key results generated from our approach and highlight key future applications and further developments of the method.
4.1 General conclusions
For all models, the predicted field residence times are rather low: fewer than 1 time step per pixel in most configurations, i.e. less than 0.2 % of simulation time, and 16 % of the maximum possible if individuals had spent all their time in the fields. The proportion of unvisited field pixels was also generally high (p>0.6). This was, however, strongly dependent on population size and movement ability (Supplement S2). This is consistent with a number of studies observing a low density of hedgerow natural enemies in the field (Al Hassan et al., 2013) and steep decreases in density from borders to the centre of the fields. Therefore, our results indicate that the low density of hedgerow natural enemies often observed inside fields may arise from the interaction between habitat quality in the fields and the movement rules of natural enemies. These results also support the hypothesis that difficulties in using naturally occurring natural enemies to control pests inside fields may be due to a spatial mismatch between natural enemies and preys, related to movement rules and habitat preferences. Indeed, our simulations predict that in most habitat configurations, natural enemies have trouble spreading into the fields where the biological control service is supposed to occur.
Overall, simulations were consistent across models and species, indicating that our predictions should be robust to intraspecific heterogeneity in movement and that we may want to reach a careful taxonomic generality. However, we observed substantial quantitative differences between insensitive and sensitive species (Fig. 4), suggesting that plans to improve field penetration by natural enemies should precisely quantify the target species' habitat specialisation. Similarly, the small but notable qualitative differences among predictions of the three models argue for a precise documentation of movement rules of the targeted species.
4.2 Mechanistic and behavioural explanations
The three models compared in this study brought substantially different results, although all concurred in predicting a concentration of individuals around good-quality habitats and provided satisfactory mechanistic explanations of the frequent field observation that individuals struggle to reach the centres of agricultural fields. Species whose behaviour corresponds to SMS results may be able to reach the highest residence times in fields in the right landscape configurations, as well as the lowest numbers of unvisited pixels. Species corresponding to the RDM model should also show high residence times and low numbers of unvisited pixels, with higher maximum scores than SMS and a higher robustness to changes in landscape configuration and movement traits (Fig. 4). In SMS, the combination of higher perceptual range and habitat selection caused a rapid convergence towards an ideal-free-like distribution, with individuals concentrating around good habitats, even being able to ignore barriers to reach a better patch, and avoiding emigration once a good destination was reached. In the RDM model, the dichotomy between routine and direct movements allowed individuals to spend more time in good habitats and rapidly cross the hostile one to find a better destination. Contrarily to SMS, individuals emigrating through a hostile habitat tended to push farther instead of turning back, but were more sensitive to barriers, leading to a more consistent spread of movements in the landscape than in SMS. Species behaving like the foraging model would be the worst case scenario, with lower residence times and higher numbers of unvisited pixels. The foraging model lacked both perceptual range and context-dependent directional persistence, which led to high concentrations in good habitats in the immediate vicinity of source patches and low ability to cross hostile areas.
The general take of this comparison among three movement strategies is that species (and behavioural sequences) with cognitively complex movement strategies will be the ones that suffer the most from ill-designed field contexts, but will also benefit more quickly from improvement in the distribution of habitat quality. Species with simple movement behaviours should show poor field penetration, irrespectively of the management implemented. The comparison between sensitive and insensitive species showed fewer differences among species than among models. However, insensitive species showed higher residence times in fields and lower numbers of unvisited pixels, suggesting that generalist species may be more interesting targets because of their lower sensitivity to habitat differences.
4.3 Managing habitat qualities of fields and surrounding habitats to improve the frequentation of fields by natural enemies
Low field frequentation and its high spatial variation may strongly impede the delivery of an efficient biological pest control service. Nevertheless, our simulations suggested that important increases in frequentation are achievable by adequately tuning habitat qualities, with the best habitat configurations providing residence times up to 86 times higher than typical habitat configurations observed in agricultural landscapes (for the foraging model; up to 84 times in SMS, up to 7 times in the RDM model; Fig. 4a) and proportions of unvisited pixels up to 3.8 times lower (for SMS; up to 1.76 times in the foraging model, up to 1.68 times in the RDM model; Fig. 4b). Indeed, the simulated maps providing the lowest residence times and the highest proportion of unvisited pixels in our simulations (Figs. 2, 3) were those corresponding to the case most frequently observed in agricultural landscapes (i.e. good hedgerow quality, low field quality, and low to intermediate GFMs).
All three models predicted higher residence times and proportions of visited pixels with a higher quality of field pixels. This prediction is very logical in view of general movement rules observed in most documented taxa: individuals tend to search for – and stay in – favourable habitats and tend to avoid hostile ones. Logically, management rules aiming at increasing the presence of natural enemies should work towards better habitat quality inside fields. Although straightforward, this guideline is nonetheless predominantly ignored in agricultural landscapes, which are dominated by homogeneous patches of heavily exploited monocultures perceived as hostile habitats by the majority of species (Altieri and Policy, 2001; Wäckers et al., 2005).
The quality of fields for the natural enemies can be increased in a number of ways: undersowing or intercropping, with other crops, or with weeds (Ratnadass et al., 2012; Schellhorn and Sork, 1997; e.g. Theunissen and Schelling, 1996). Such an increase in plant species diversity may provide alternative food sources to natural enemies (Tylianakis et al., 2004) and attractive visual or olfactive stimuli (Cook et al., 2007), and changes in vegetation structure may provide shelters for alternative hosts and preys (Bianchi et al., 2006) and shelters for natural enemies (Mailloux et al., 2010). Conversely, mixtures of crop cultivars are unlikely to provide any effect on individual movement, as they rarely result in structural, visual, or olfactive heterogeneity (Ratnadass et al., 2012). The low permeability of fields also suggests decreasing the grain of the landscape, which would make it more likely for movements from hedgerows to reach the centre of the fields (Baguette and Van Dyck, 2007).
Peripheral, semi-natural elements are frequently advocated as a crucial support to conservation biological control (Bianchi et al., 2006) by providing refuges from the perturbations of farming practices (Bianchi et al., 2006; Griffiths et al., 2008). In our simulations, however, the good quality of hedgerows had a negative effect on the field frequentation. Of course, it would make little sense to argue for hostile hedgerows (especially when those are to be the source habitat of naturally occurring natural enemies), but based on this result we argue that management efforts should focus on increasing the quality of fields rather than improving hedgerows, as far as movement is concerned.
If the natural enemy is a species whose life cycle includes landscape complementation (Dunning et al., 1992), it would be interesting to make hedgerows and fields differentially attractive and repulsive during different parts of the life cycle, so that individuals reproducing in hedgerows tend to move preferentially towards the field during foraging phases.
Grassy field margins are frequently set up in agricultural landscapes, with anticipated positive effects on biological control by providing intermediary habitats that would favour movements of natural enemies coming from hedgerows and by providing floral resources that attract natural enemies in the vicinity of fields (Dennis and Fry, 1992; Denys and Tscharntke, 2002; Al Hassan et al., 2013; Olson and Wäckers, 2007). Here, we suggest that only GFMs with average habitat quality may provide beneficial effects, while GFMs with bad quality act as a barrier and GFMs with good quality attract individuals out of the field (Fig. 3). This result may provide an explanation mechanism for the frequent hiccoughs in the implementation of GFMs for biological control, with predator density decreasing strongly with distance (Denys and Tscharntke, 2002), being lower in the vicinity of the GFM (Broekhuizen et al., 1986) or reacting inconsistently with spatial context and GFM quality (Haaland and Bersier, 2010; Al Hassan et al., 2013).
Push–pull strategies are frequently integrated with biological control in order to lure pests away from – and natural enemies towards – cultivated crops (Cook et al., 2007) by means of attractants and repellents. Our simulations suggest that, even in the absence of a specific push–pull strategy, its underlying principle applies to a wide range of configurations of arable fields. The interactions between neighbour habitats being perceived as hostile and beneficial can strongly affect the presence of natural enemies in the arable field, by drawing them out or preventing them from entering (Figs. 3 and 4).
4.4 Limitations and perspectives
The biological control service is based on more than just individual movements, and every underlying ecological process should be considered concurrently in order to provide meaningful guidelines. Our study design did not include inter-individual or interspecific interactions, while prey abundance and competition are important clues used in the evaluation of a given habitat. Future developments should also explore the beneficial effects of an increase in heterogeneity of the field structure: structural elements such as intra-field corridors or stepping stones could probably help, as our results suggest that individuals struggle to reach the centre of fields and may benefit from a careful management of the distribution of habitat qualities.
The restricted hedgerow-centred point of view that we purposely chose here could be substantially expanded, by considering species that originate from GFMs or from each habitat with a variable proportion. The same approach could also be used to search for the best configurations of habitat to keep pests out of the fields, in combination with other techniques such as trap crops (Shelton and Badenes-Pérez, 2006).
Data are available from a free public repository at https://doi.org/10.13140/RG.2.2.13613.13288.
The supplement related to this article is available online at: https://doi.org/10.5194/we-19-15-2019-supplement.
TD performed simulations and analysis and helped write the paper. BC and CL helped write the paper.
The authors declare that they have no conflict of interest.
We warmly thank the three reviewers and Matthias Foellmer for their
beneficial comments that helped to improve the quality of this article. B.
Collard was supported by INRA (French National Institute for Agricultural
Research, EA division), by CIRAD (French Agricultural Research Centre for
International Development), and by the STRADIV project (no. 1504-003)
funded by the Agropolis Foundation, France.
Edited by: Matthias Foellmer
Reviewed by: three anonymous referees
Al Hassan, D., Georgelin, E., Delattre, T., Burel, F., Plantegenest, M., Kindlmann, P., and Butet, A.: Does the presence of grassy strips and landscape grain affect the spatial distribution of aphids and their carabid predators?, Agric. For. Entomol., 15, 24–33, 2013.
Altieri, M. A. and Policy, S.: The Nature and Function of Biodiversity in Agriculture, Ecol., 5–8, 2001.
Arrignon, F., Deconchat, M., Sarthou, J. P., Balent, G., and Monteil, C.: Modelling the overwintering strategy of a beneficial insect in a heterogeneous landscape using a multi-agent system, Ecol. Modell., 205, 423–436, https://doi.org/10.1016/j.ecolmodel.2007.03.006, 2007.
Aviron, S., Kindlmann, P., and Burel, F.: Conservation of butterfly populations in dynamic landscapes: The role of farming practices and landscape mosaic, Ecol. Modell., 205, 135–145, 2007.
Baguette, M. and Van Dyck, H.: Landscape connectivity and animal behaviour: functional grain as a key determinant for dispersal, Landsc. Ecol., 22, 1117–1129, https://doi.org/10.1007/s10980-007-9108-4, 2007.
Barbosa, P.: Conservation biological control, Academic Press, 1998.
Bartumeus, F., da Luz, M. G. E., Viswanathan, G. M., and Catalan, J.: Animal Search Strategies: a Quantitative Random-Walk Analysis, Ecology, 86, 3078–3087, https://doi.org/10.1890/04-1806, 2005.
Bell, W. J.: Searching behaviour. The behavioural ecology of finding resources, Chapman & Hall, London., 1991.
Bianchi, F. J. J. A., Booij, C. J. H., and Tscharntke, T.: Sustainable pest regulation in agricultural landscapes: A review on landscape composition, biodiversity and natural pest control, P. Roy. Soc. B, 273, 1715–1727, https://doi.org/10.1098/rspb.2006.3530, 2006.
Bocedi, G., Palmer, S. C. F., Pe'er, G., Heikkinen, R. K., Matsinos, Y. G., Watts, K., and Travis, J. M. J.: RangeShifter: a platform for modelling spatial eco-evolutionary dynamics and species' responses to environmental changes, edited by R. Freckleton, Methods Ecol. Evol., 5, 388–396, https://doi.org/10.1111/2041-210X.12162, 2014.
Bonte, D., Van Dyck, H., Bullock, J. M., Coulon, A., Delgado, M., Gibbs, M., Lehouck, V., Matthysen, E., Mustin, K., Saastamoinen, M., Schtickzelle, N., Stevens, V. M., Vandewoestijne, S., Baguette, M., Barton, K., Benton, T. G., Chaput-Bardy, A., Clobert, J., Dytham, C., Hovestadt, T., Meier, C. M., Palmer, S. C. F., Turlure, C., and Travis, J. M. J.: Costs of dispersal, Biol. Rev. Camb. Philos. Soc., 87, 290–312, https://doi.org/10.1111/j.1469-185X.2011.00201.x, 2012.
Börger, L., Dalziel, B. D., and Fryxell, J. M.: Are there general mechanisms of animal home range behaviour? A review and prospects for future research, Ecol. Lett., 11, 637–650, https://doi.org/10.1111/j.1461-0248.2008.01182.x, 2008.
Broekhuizen, S., C. A., H., van't Maaskamp, F., and Pauwels, T.: The importance of hedgerows for leading migrating badgers Meles meles, Lutra, 29, 54–65, 1986.
Burel, F., Baudry, J., Butet, A., Clergeau, P., Delettre, Y., Le Coeur, D., Dubs, F., Morvan, N., Paillat, G., Petit, S., Thenail, C., Brunel, E., and Lefeuvre, J. C.: Comparative biodiversity along a gradient of agricultural landscapes, Acta Oecologica-International J. Ecol., 19, 47–60, 1998.
Clobert, J., Danchin, E., Dhondt, A. A., and Nichols, J. D.: Dispersal, Oxford University Press, Oxford., 2001.
Codling, E. A., Plank, M. J., and Benhamou, S.: Random walk models in biology, J. R. Soc. Interface, 5, 813–834, https://doi.org/10.1098/rsif.2008.0014, 2008.
Conradt, L., Zollner, P. A., Roper, T. J., Frank, K., and Thomas, C. D.: Foray search: An effective systematic dispersal strategy in fragmented landscapes, Am. Nat., 161, 905–915, 2003.
Cook, S. M., Khan, Z. R., and Pickett, J. A.: The Use of Push-Pull Strategies in Integrated Pest Management, Annu. Rev. Entomol., 52, 375–400, https://doi.org/10.1146/annurev.ento.52.110405.091407, 2007.
Coombes, D. S. and Sothertons, N. W.: The dispersal and distribution of polyphagous predatory Coleoptera in cereals, Ann. Appl. Biol., 108, 461–474, https://doi.org/10.1111/j.1744-7348.1986.tb01985.x, 1986.
Coulon, A., Aben, J., Palmer, S. C. F., Stevens, V. M., Callens, T., Strubbe, D., Lens, L., Matthysen, E., Baguette, M., and Travis, J. M. J.: A stochastic movement simulator improves estimates of landscape connectivity, Ecology, 96, 2203–2213, https://doi.org/10.1890/14-1690.1, 2015.
Delattre, T., Burel, F., Humeau, A., Stevens, V. M., Vernon, P., and Baguette, M.: Dispersal mood revealed by shifts from routine to direct flights in the meadow brown butterfly Maniola jurtina, Oikos, 119, 1900–1908, https://doi.org/10.1111/j.1600-0706.2010.18615.x, 2010a.
Delattre, T., Pichancourt, J.-B., Burel, F., and Kindlmann, P.: Grassy field margins as potential corridors for butterflies in agricultural landscapes: A simulation study, Ecol. Modell., 221, 370–377, https://doi.org/10.1016/j.ecolmodel.2009.10.010, 2010b.
Delattre, T., Vernon, P., and Burel, F.: An Agri-environmental scheme enhances butterfly dispersal in European agricultural landscapes, Agric. Ecosyst. Environ., 166, 102–109, 2013.
Delattre, T.: Data from the paper “Keep your enemies closer: enhancing biological control through individual movement rules to retain natural enemies inside the field”, Web Ecol., 18, 1–17, https://doi.org/10.5194/we-18-1-2018, 2018.
Dennis, P. and Fry, G. L. A.: Field margins: can they enhance natural enemy population densities and general arthropod diversity on farmland?, Agric. Ecosyst. Environ., 40, 95–115, 1992.
Denys, C. and Tscharntke, T.: Plant-insect communities and predator-prey ratios in field margin strips, adjacent crop fields, and fallows, Oecologia, 130, 315–324, https://doi.org/10.1007/s004420100796, 2002.
Dover, J. W. and Fry, G. L. A.: Experimental simulation of some visual and physical components of a hedge and the effects on butterfly behaviour in an agricultural landscape, Entomol. Exp. Appl., 100, 221–233, 2001.
Dunning, J., Danielson, B., and Pulliam, H.: Ecological processes that affect populations in complex landscapes, Oikos, 1992.
Ernoult, A., Vialatte, A., Butet, A., Michel, N., Rantier, Y., Jambon, O., and Burel, F.: Grassy strips in their landscape context, their role as new habitat for biodiversity, Agric. Ecosyst. Environ., 166, 15–27, https://doi.org/10.1016/j.agee.2012.07.004, 2013.
Fahrig, L., Baudry, J., Brotons, L., Burel, F. G., Crist, T. O., Fuller, R. J., Sirami, C., Siriwardena, G. M., and Martin, J.-L.: Functional landscape heterogeneity and animal biodiversity in agricultural landscapes, Ecol. Lett., 14, 101–112, https://doi.org/10.1111/j.1461-0248.2010.01559.x, 2010.
Griffiths, G. J. K., Holland, J. M., Bailey, A., and Thomas, M. B.: Efficacy and economics of shelter habitats for conservation biological control, Biol. Control, 45, 200–209, https://doi.org/10.1016/j.biocontrol.2007.09.002, 2008.
Grimm, V. and Railsback, S. F.: Individual-based Modeling and Ecology, Princeton University Press, Princeton, 2005.
Grimm, V., Berger, U., Bastiansen, F., Eliassen, S., Ginot, V., Giske, J., Goss-Custard, J., Grand, T., Heinz, S. K., and Huse, G.: A standard protocol for describing individual-based and agent-based models, Ecol. Modell., 198, 115–126, https://doi.org/10.1016/j.ecolmodel.2006.04.023, 2006.
Grimm, V., Berger, U., DeAngelis, D. L., Polhill, J. G., Giske, J., and Railsback, S. F.: The ODD protocol: A review and first update, Ecol. Modell., 221, 2760–2768, https://doi.org/10.1016/j.ecolmodel.2010.08.019, 2010.
Haaland, C. and Bersier, L.-F.: What can sown wildflower strips contribute to butterfly conservation?: an example from a Swiss lowland agricultural landscape, J. Insect Conserv., 15, 301–309, https://doi.org/10.1007/s10841-010-9353-8, 2010.
Haddad: Corridor use predicted from behaviors at habitat boundaries, Am. Nat., 153, 215–227, https://doi.org/10.2307/2463582, 1999.
Hamilton, N.: ggtern: An Extension to “ggplot2”, for the Creation of Ternary Diagrams, 2017.
Human Rights Council: Report of the Special Rapporteur on the right to food, 2017.
Kindlmann, P., Aviron, S., Burel, F., and Ouin, A.: Can the assumption of a non-random search improve our prediction of butterfly fluxes between resource patches?, Ecol. Entomol., 29, 447–456, https://doi.org/10.1111/j.0307-6946.2004.00614.x, 2004.
Kindlmann, P., Aviron, S., and Burel, F.: When is landscape matrix important for determining animal fluxes between resource patches?, Ecol. Complex., 2, 150–158, https://doi.org/10.1016/j.ecocom.2004.11.007, 2005.
Kleijn, D., Baquero, R. A., Clough, Y., Díaz, M., De Esteban, J., Fernández, F., Gabriel, D., Herzog, F., Holzschuh, A., Jöhl, R., Knop, E., Kruess, A., Marshall, E. J. P., Steffan-Dewenter, I., Tscharntke, T., Verhulst, J., West, T. M., and Yela, J. L.: Mixed biodiversity benefits of agri-environment schemes in five European countries, Ecol. Lett., 9, 243–254, https://doi.org/10.1111/j.1461-0248.2005.00869.x, 2006.
Kremen, C., Williams, N. M., Aizen, M. A., Gemmill-Herren, B., LeBuhn, G., Minckley, R., Packer, L., Potts, S. G., Roulston, T., Steffan-Dwenter, I., Vasquez, D. P., Winfree, R., Adams, L., Crone, E. E., Greenleaf, S. S., Keitt, T. H., Klein, A. M., Regetez, J., and Ricketts, T. H.: Pollination and other ecosystem services produced by mobile organisms: a conceptual framework for the effects of land-use change, Ecol. Lett., 10, 299–314, 2007.
Long, S. J.: Regression models for categorical and limited dependent variables, Sage, 1997.
Lys, J.-A., Zimmermann, M., and Nentwig, W.: Increase in activity density and species number of carabid beetles in cereals as a result of strip-management, Entomol. Exp. Appl., 73, 1–9, https://doi.org/10.1111/j.1570-7458.1994.tb01833.x, 1994.
Mailloux, J., Le Bellec, F., Kreiter, S., Tixier, M.-S., and Dubois, P.: Influence of ground cover management on diversity and density of phytoseiid mites (Acari: Phytoseiidae) in Guadeloupean citrus orchards, Exp. Appl. Acarol., 52, 275–290, https://doi.org/10.1007/s10493-010-9367-7, 2010.
Malézieux, E., Crozat, Y., Dupraz, C., Laurans, M., Makowski, D., Ozier-Lafontaine, H., Rapidel, B., De Tourdonnet, S., and Valantin-Morison, M.: Mixing plant species in cropping systems: Concepts, tools and models: A review, in Sustainable Agriculture, 329–353, Springer Netherlands, Dordrecht, 2009.
Mitchell, M. S. and Powell, R. A.: A mechanistic home range model for optimal use of spatially distributed resources, Ecol. Modell., 177, 209–232, https://doi.org/10.1016/j.ecolmodel.2004.01.015, 2004.
Olson, D. M. and Wäckers, F. L.: Management of field margins to maximize multiple ecological services, J. Appl. Ecol., 44, 13–21, 2007.
Palmer, S. C. F., Coulon, A., and Travis, J. M. J.: Introducing a “stochastic movement simulator” for estimating habitat connectivity, Methods Ecol. Evol., 2, 258–268, https://doi.org/10.1111/j.2041-210X.2010.00073.x, 2011.
Papaïx, J., Touzeau, S., Monod, H., and Lannou, C.: Can epidemic control be achieved by altering landscape connectivity in agricultural systems?, Ecol. Modell., 284, 35–47, https://doi.org/10.1016/j.ecolmodel.2014.04.014, 2014.
Pulido, F. and Berthold, P.: Current selection for lower migratory activity will drive the evolution of residency in a migratory bird population, P. Natl. Acad. Sci. USA, 107, 7341–7346, https://doi.org/10.1073/pnas.0910361107, 2010.
Ratnadass, A., Fernandes, P., Avelino, J., and Habib, R.: Plant species diversity for sustainable management of crop pests and diseases in agroecosystems: a review, Agron. Sustain. Dev., 32, 273–303, https://doi.org/10.1007/s13593-011-0022-4, 2012.
R Core Team: R: a Language and Environment for Statistical computing, https://doi.org/10.1007/978-3-540-74686-7, 2011.
Root-Bernstein, M. and Jaksic, F. M.: Making research relevant? Ecological methods and the ecosystem services framework, Earth's Futur., 5, 664–678, https://doi.org/10.1002/2016EF000501, 2017.
Schellhorn, N. A. and Sork, V. L.: The impact of weed diversity on insect population dynamics and crop yield in collards, Brassica oleraceae (Brassicaceae), Oecologia, 111, 233–240, https://doi.org/10.1007/s004420050230, 1997.
Shelton, A. M. and Badenes-Pérez, F. R.: Concepts and applications of trap cropping in pest management, Annu. Rev. Entomol., 51, 285–308, https://doi.org/10.1146/annurev.ento.51.110104.150959, 2006.
Simberloff, D. and Stiling, P.: Risks of species introduced for biological control, Biol. Conserv., 78, 185–192, https://doi.org/10.1016/0006-3207(96)00027-4, 1996.
Stevens, V. M., Wesselingh, R. A., and Baguette, M.: Demographic processes in a small, isolated population of natterjack toads (Bufo calamita) in southern Belgium, Herpetol. J., 13, 59–67, 2003.
Thenail, C. and Baudry, J.: Variation of farm spatial land use pattern according to the structure of the hedgerow network (bocage) landscape: a case study in northeast Brittany, Agric. Ecosyst. Environ., 101, 53–72, https://doi.org/10.1016/s0167-8809(03)00199-3, 2004.
Theunissen, J. and Schelling, G.: Pest and disease management by intercropping: Suppression of thrips and rust in leek, Int. J. Pest Manag., 42, 227–234, https://doi.org/10.1080/09670879609372000, 1996.
Tisue, S. and Wilensky, U.: Netlogo: A simple environment for modeling complexity, Vol. 21, 16–21, Boston, MA., 2004.
Tylianakis, J. M., Didham, R. K., and Wratten, S. D.: Improved fitness of aphid parasitoids receiving resource subsidies, Ecology, 85, 658–666, https://doi.org/10.1890/03-0222, 2004.
Van Dyck, H. and Baguette, M.: Dispersal behaviour in fragmented landscapes: Routine or special movements?, Basic Appl. Ecol., 6, 535–545, https://doi.org/10.1016/j.baae.2005.03.005, 2005.
Vasseur, C., Joannon, A., Aviron, S., Burel, F., Meynard, J.-M., and Baudry, J.: The cropping systems mosaic: How does the hidden heterogeneity of agricultural landscapes drive arthropod populations?, Agric. Ecosyst. Environ., 166, 3–14, 2013.
Veres, A., Petit, S., Conord, C., and Lavigne, C.: Does landscape composition affect pest abundance and their control by natural enemies? A review, Agr. Ecosyst. Environ., 166, 110–117, https://doi.org/10.1016/J.AGEE.2011.05.027, 2013.
Wäckers, F. L., van Rijn, P. C. J., and Bruin, J.: Plant-Provided Food for Carnivorous Insects, edited by: Wäckers, F. L., van Rijn, P. C. J., and Bruin, J., Cambridge University Press., 2005.